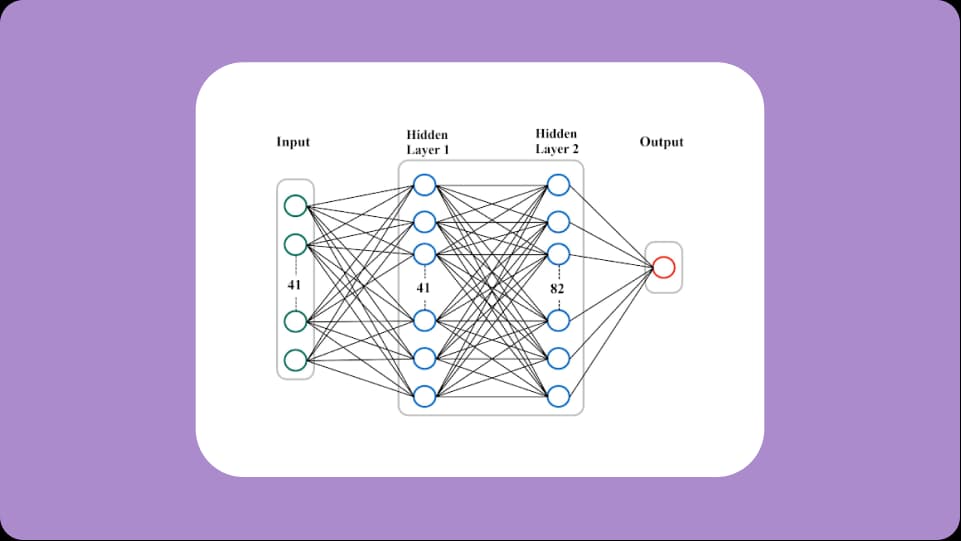
- Data Science
- Python
- PyTorch
- PyCaret
- SKLearn
- Numpy
- Pandas
Appendicular Lean Mass Prediction Using PyTorch
Introduction
In the summer of 2022, a team of students and researchers from the LSU Math Department embarked on a captivating journey funded by Pennington Biomedical Research Center. The goal of this group was to enhance the findings of one of Pennington's research papers. We aimed to elevate the accuracy of predicting Appendicular Lean Mass (ALM) using neural networks, a departure from the original study's approach involving least absolute shrinkage and LASSO regression.
The Team
The team consisted of around five undergraduates and two graduate students led by a postdoc and a professor from the department. Several members of the team had additional concentrations in related fields like computer science, engineering, and biology.
Methodology
-
Visualizing and understanding the Data: Employing visualization tools, such as Matplotlib and Seaborn, helped us gain valuable insights into the dataset.
-
Data Cleaning and Anomaly Detection: PyCaret was our primary tool in streamlining anomaly detection and data cleaning processes.
-
Building the Network with PyTorch: We constructed a neural network using PyTorch. We almost immediately found promising results, even before optimizing the mode.
-
Hyperparameter Tuning: We fine-tuned the model's predictive performance through meticulous hyperparameter optimization.
Results and Achievements
Continuing our efforts into the Fall, our work received recognition as a featured poster during Obesity Week.